Abstract
The present study considers cloud computing and its integration into the supply chain. The history of supply chain management demonstrates the discipline’s interest in innovation, computerisation and automation of key supply chain processes has led to increased efficiency and profit. Currently, the idea of using cloud technologies is at the centre of discussions as its potential to further enhance the performance is considered. The aim of this work is to review the existing scholarship on the topics of cloud computing in the supply chain, including such methods, services and systems as big data, artificial intelligence and the Internet-of-Things.
The research method is data synthesis, which is complete using thematic analysis of scholarly article and case studies pertaining to the theme of the discussion. The results of the investigation revealed that the literature on cloud computing considers it a vital step in improving supply chain management. The integration of cloud-based services is a part of Industry 4.0, although this process is only on its first stage. Cloud computing offers many benefits to the supply chain, increasing productivity and reducing the rate of human errors. Nonetheless, it also has some risks since it is a relatively new concept that still has many vulnerabilities.
Acknowledgements
The author wishes to express thanks to all those who have assisted and advised during this project, with particular mention of:
Introduction
Background to the Research
The history of supply chain management demonstrates that this discipline has to adhere to societal changes to ensure stability and growth. The roots of supply chain management go into the beginning of the twentieth century, when the idea of industrial engineering was developed (Monczka et al. 2015). The value of analytics in business and the inclusion of logistics as a part of any industry evolved further to take a significant place in supply chain management.
In the 1950s, the main focus of the industry’s research was mechanisation – the integration of technology and devices to improve labour-intensive processes and assist people in material handling and transportation (MacCarthy et al. 2016). Therefore, manager began paying increased attention to such issues as warehouse design, transportation modes and effective unit load (Mangan and Lalwani 2016). However, the major part of supply chain management – record keeping and updating – was performed manually, which inevitably led to errors, notably if a company’s workload increased beyond people’s capabilities.
As a result of mechanisation, the efficiency of warehouses, transportation and material handling was reformed. However, all operations were still performed and managed by people who had to work with continuously increasing amounts of data. One of the steps that supply chain managers had to complete in the previous decades was computerisation – automation of processes, the use of computers to calculate, track and document all operations in the chain (Monczka et al. 2015).
In 1970 and 1980s, the newly designed technology was investigated to find ways to use its abilities to serve industrial production (MacCarthy et al. 2016). The sphere of supply chain management changed further with the development of portable computers, handheld tablets, smartphones and other devices. Moreover, the rising idea of globalised businesses contributed to other issues, broadening the reach of companies and their need to work with more organisations and track rising quantities of materials (Christopher 2016). The growth of manufacturing and the establishment of a global competitive market urged the sphere of supply chain management to innovate continuously.
Currently, the products of the previous research can be seen in the majority of organisations. Supply chain management has integrated other developments, including the Internet, broadband communications, and automatic record-keeping, into its functioning (Stevens and Johnson 2016).
Nevertheless, the growing amount of information that a business has to analyse and store on a daily basis creates new problems and poses a question of what can be done to improve the discipline further. At the present time, managers are considering another solution to enhancing their performance – cloud computing. The development of cloud storage and its utilisation in various fields reveals broad possibilities for business. Thus, it is necessary to research the history and prospects of this field and see how it can be and was introduced to the sphere of supply chain management.
The investigation of previous steps taken to innovate supply chain management can show which aspects of the business need to evolve in order for organisations to follow the trend and stay prosperous and efficient. While the initial steps to the development of this discipline are outlined in the background of the research, a closer investigation of the chain’s computerisation is required. A mentioned above, initially, all transactions and record-keeping procedures were paper-based.
This type of data processing was not only time-consuming, but also prone to human error (Christopher 2016). In the 1960s, the logistics industry was integrating with supply chain management, but the technology was limited to put theoretical formulas into practice. In the 1980s, personal computers were introduced to the world, and managers and business researchers quickly discovered the devices’ potential. Planners, who previously only worked with written records, received access to a device that could handle a more significant amount of information and present it is a visually understandable way (MacCarthy et al. 2016). The development of computers was a step that contributed to the growth of supply chain research.
Previously explored topics of process automation and planning efficiency were applied in the field of business technology. In 1983, an improved Manufacturing Resource Planning (MRP II) was introduced to the industry (Tao et al. 2018). Such concepts as sales and operations planning, master scheduling, and rough-cut capacity planning were implemented in the discipline (Tao et al. 2018). The United States’ companies invested in hardware and software to manage data, and the supply chain’s globalisation continued to expand.
Computers allowed businesses to manage operations in other countries, and offshore manufacturing gained popularity due to availability and cost (MacCarthy et al. 2016). The access to more data gave companies an opportunity to understand the factors that improved efficiency and logistics of the supply chain. It also urged them to innovate further, using strategies to increase computer integration. As a result, such systems as the Advanced Planning and Scheduling (APS) software were developed.
At the beginning of the twenty-first century, another part of the computerisation process started to develop – the increase in connectivity. The Internet drastically changed people’s view of information sharing, lowering costs and raising speeds of data transfer (Hong et al. 2017). While portable computers before the Internet could store data and perform calculations, information transfer was still slow and unsafe. The addition of the Internet offered to eliminate these issues while cutting expenses as well.
Looking at the process of computerisation that occurred in the supply chain management in the 1980s-2000s, one can see which drivers were the most significant. First of all, the need to store growing amounts of data contributed to new strategies of increasing memory and optimising information cataloguing. Second, globalisation incentivised companies to create channels of communication that would be fast and secure. Finally, all data had to be consistent in quality and speed of distribution, thus asking for efficient channels for information dissemination, interconnected databases and a system that can handle the rising needs of supply chain members as well as customers.
In the 1970s, the US government worked on the version of the Internet called the Advanced Research Project Administration (ARPANET) (Marinescu 2017). This system’s purpose was to share computer-based resources between users, regardless of their location. In the 1980s, the National Science Foundation tried to connect supercomputers and design a network based on these sites. At the same time, first commercial Internet Service Providers started to emerge (Marinescu 2017). In the end, the rise of the Internet followed out of these efforts, and the World Wide Web entered people’s lives.
Nonetheless, cloud computing as a service began appearing later, as more and more companies offered their services using the Internet. Cloud computing was not created for the sole purpose of serving businesses. Its development contributed to the lives of all Internet users, leading to its wide implementation (Hashem et al. 2015; Marinescu 2017). The term ‘cloud computing’ appeared as early as 1996, being used in an internal company document of Compaq (Marinescu 2017).
However, the idea of a ‘cloud’ in relation to remote storage options had been discussed even before that, although the name of the concept was ‘distributed’ computing (Marinescu 2017). One of the significant contributors to the sphere of cloud computing was Salesforce.com – a website that launched in 1999 and worked to deliver cloud-based enterprise applications (Marinescu 2017). Businesses could access the website, purchase software and download it on the Internet, without leaving the office.
Currently, this type of product is known as ‘Software-as-a-Service’ (SaaS), and it is a major part of cloud computing (Diaby and Rad 2017). The next milestone for cloud computing happened in 2002 when Amazon launched its Web Services – a collection of cloud-based services that customers could purchase and use instantly. In 2006, Amazon continued to contribute to this sphere by opening the Elastic Compute Cloud (Marinescu 2017). It was a web service for small businesses that would allow them to rent and use computers for their company operations. All applications for the devices were supplied using Amazon’s cloud, and this framework was later used by other services, including streaming and data-sharing platforms.
The first widely known Platform-as-a-Service (PaaS) was developed by Google. In 2008, the company launched Google App Engine (GAE), which gave developers an opportunity to host their own web applications on the Google platform (Marinescu 2017). The information and data related to these apps were based in and managed by the business’ own data centres. Currently, the alternative for GAE is Google Apps – a platform that hosts many applications from developers and makes them available for users with different traffic requirements. Later, a combination of PaaS and SaaS was offered by Microsoft on this platform, Azure (Marinescu 2017).
Azure could also be considered an Infrastructure-as-a-Service (IaaS) – a self-service for accessing storage, programmes, networks and other products (Marinescu 2017). As more and more organisations entered the market for cloud-computing services as a way of supplying and storing software for customers, other business segments began to think about how they could use cloud computing to their advantage.
The Subject
The subject of this study is the integration of cloud computing in relation to supply chain management practices. The concept of cloud computing is relatively new for all industries as well as individuals using it in their daily life. However, considering the speed of innovation that increased after the Internet was developed, one may see why cloud computing is a step on which supply chain management researchers are focusing their attention.
The choice to investigate cloud computing as a valuable addition to supply chains is essential today since many companies become increasingly reliant on technology and process automation. Manual labour and in-person processes are a vital part of all business operations, but the globalised market can no longer be sustained utilising only human-to-human and human-to-machine interactions (Lamba and Singh 2017). The implementation of complex technology-based operations is the next step that will be considered in this research.
The Aim
The main aim of the study is to analyse the current and potential contribution of cloud computing to all elements of the supply chain. The existing research focuses on computerisation, and a fewer number of works consider the possibilities of cloud computing in the supply chain. Cloud computing in business offers another view of technology as not just an assistant to a human worker, but also as a fundamental part of all processes (Jede and Teuteberg 2015).
To understand the role of cloud computing in the performance of businesses, it is necessary to see how it was developed and how it elevated the sphere of computer technology. Its contribution to supply chain management operations, potential risks of using big data, benefits of establishing a software-focused chain and similar questions will be considered by using existing scholarship as well.
Therefore, the first objective of this study is to investigate the history of supply chain management innovation as well as the development of cloud computing. Next, the second objective is to review how cloud computing is being applied in the supply chain. The third objective includes an analysis of expectations, benefits, and risks of increasing the use of cloud computing based on the synthesis of available evidence.
Review of Literature
Cloud Computing Development
In the most recent years, major companies started offering cloud services for many purposes, entering the era of cloud-based operating. Currently, people are able to access software and media that is shared and stored in the cloud (Kushida et al. 2015). One can also store any type of files, including private and business documents, photos or videos. Moreover, a cloud can run an entire infrastructure (IaaS) for an organisation, allowing users to manage their middleware and operating system (OS).
The growing influence of cloud computing on everyday access to data and software, especially the frameworks of SaaS, PaaS and IaaS, was explored in relation to business by Shee et al. (2018). Some companies already utilise these services on a smaller scale, moving their documents to the cloud or sharing information using Internet-based applications. Nevertheless, to understand why cloud computing may offer new possibilities to supply chain management, one has to look at influential innovations that this type of service provision includes.
All technological advancements in the sphere of cloud computing fit into the framework of Industry 4.0 – a part of the fourth industrial revolution. This idea was first introduced in 2011 as an element of the new German economic policy (Roblek et al. 2016). Later, the term “Industry 4.0” became associated with the world’s continuous development of technology.
This period of innovation is guided by such subsets of technology as robotics, artificial intelligence (AI), wireless technologies of the fifth generation (5G), autonomous vehicles, and cyber-physical systems (QIN et al. 2016). Here, cloud computing encompasses several of the mentioned above concepts, providing a base for AI and automation and combining wireless technologies with data gathering and sharing (Liao et al. 2017). Therefore, it is vital to review the subject if cloud computing and its elements through the lens of Industry 4.0.
For businesses, the concept of Industry 4.0 includes such ideas as smart manicuring and industrial Internet of Things. Smart factories with autonomous vehicles transporting goods and managing machinery without human intervention is a part of the vision for the future. Across the value chain, the implementation of cyber-physical systems is suggested to create relationships between people and machines which will be characterised by humans’ limited interaction with small processes and mistakes. Minor inconsistencies and errors are to be covered by computers’ self-learning based on AI implementation.
The achievement of Industry 4.0 is rooted in the alignment of an organisation with four principles – interoperability, technical assistance, information transparency and decentralised decision making (Thames and Schaefer 2016). Interoperability, otherwise called interconnection, implies that machines and people will work in a single system and share information using the Internet of Things (IoT).
Here, the role of people will not be to guide the machines but to work alongside computers, sensors and devices powered by AI. Information transparency suggests that all data available through the internet will help people to make informed decisions. The principle of technical assistance addresses the machines’ ability to support humans in areas where one’s physical and intellectual powers are insufficient or can be enhanced by computers. Lastly, the expected level of computers’ autonomy will lead to the creation of decentralized systems not guided by one person. As can be seen, the elements of the revolution empowered by cloud computing such as big data, IoT and AI contribute to the establishment of Industry 4.0.
Cloud Computing and Supply Chain Management: Existing Examples and Spheres of Use
Barcoding and the Radio-Frequency Identification (RFID)
One of the most prominent inventions in the technology-focused supply chain research was the Radio-Frequency Identification (RFID) technology. Before the invention of RFIDs, barcodes were used as the primary method of representing data in a form that a machine can read from a marked object (Zhong et al. 2015). Barcode readers read tags that were placed directly in front of them, collecting data from each product one by one.
This system, while faster than a human-operated and paper-based system, had many limitations, especially for warehouses and facilities with large quantities of objects to scan. In the 1980s, the concept of using radio frequencies to scan information was patented, and RFID tags slowly entered industries and the supply chain (Zhong et al. 2015). Currently, the use of RFID technology in the supply chain is essential to warehousing and transportation as it allows for fast and easy tracking of the goods and equipment.
The benefits of RFID over barcoding demonstrate the progress that wireless technology encouraged in the supply chain. Digital data encoded in specific RFID tags attached to transportation pallets is decoded by a reader using radio waves (Biswal et al. 2018). The implementation of this tagging system by distribution centres provided companies with an opportunity to track materials and update databases in real-time by implementing wireless technology. In contrast to barcodes, RFID tags can be read from a longer distance as they operate on the principle of “near-field” – the tag has to be in the range of the scanner to be recognized (Kang et al. 2015).
Moreover, RFID technology can record more data on one tag, giving businesses an ability to monitor the flow of objects, the timestamps of movements and general information about them (Biswal et al. 2018). Finally, the speed of reading RFID tags is much higher than that of barcodes, and multiple RFID tags can be read at once, further increasing the efficiency of businesses with this technology.
The introduction of RFID technology showed that computerisation was a necessary step for elevating the performance of the supply chain. From that point on, the use of technology in supply chain management continued to grow. The Internet’s speed was increasing, and computers became smaller and more efficient in not only storing data but also making calculations and sophisticated analyses for which a person would require significantly more time to complete (Waluyo 2019). Currently, the use of RFID technology is being integrated within the framework of cloud computing to empower the growing IoT systems (Kang et al., 2015).
Tags with information act as devices that make each marked object recognisable to machines operating in the supply chain. As a result, the information collected using RFID and transported to the cloud becomes one of the pillars of Industry 4.0. and its principle of interoperability.
E-commerce
The sphere of e-commerce further contributed to the supply chain members’ drive to improve the performance of computerised systems. People’s ability to buy products on the Internet and the rise in small items’ delivery changed the way consumers viewed products’ accessibility. Thus, a sphere of e-commerce logistics was introduced as a segment of the discipline. Here, the focus was placed on quick delivery and effective database searching (Yu et al. 2016) Companies, willing to invest in online stores, have to create a framework that supports both online and offline purchases, as well as developing relations with other supply chain participants to deliver smooth service without delays (Almarabeh and Majdalawi 2019).
Now, e-commerce takes up a significant part of all retail operations, and its integration of cloud computing services bolsters its appeal to customers and changes how it operates with the supply chain. As mentioned previously, cloud computing increases the scalability of software and hardware that businesses can use. By operating in the cloud, companies save resources and utilize the memory storage that does not put additional pressure on their finances (Almarabeh and Majdalawi 2019).
Moreover, the speed of such operations is also becoming quicker every year with cloud computing research increasing the load that businesses are able to handle. Similar to offline-based businesses, e-commerce gets the same benefits from the cloud, with the addition of placing its services in the same system for customers to use. Thus, the information collected by e-commerce businesses enters the pool of information used in big data analytics.
Big Data
The term ‘big data’ describes not the information itself but an approach to its analysis. Data collected from various sources that are available to the public is stored on servers that are connected through the internet. People and devices can use this data for their projects – for example, people’s choices, clicks, search options and preferences are used in analysing populations and small groups. Nonetheless, with time, the amount of data that exists on the Internet in public access became too large and complex to be examined using traditional processing methods (Hashem et al. 2015). This event caused the rise of big data – a strategy that takes the information from cloud services and uses it in machine-guided calculations, providing a broad outlook on the state of an industry or concept.
The process of collecting, analysing, and synthesising information has been a part of business research for decades. It is a necessary step in understanding clients, planning budgets, predicting expenses as well as monitoring the quality products. The use of big data has been adopted by different business spheres, including marketing and advertising, as well as feedback and quality evaluation (Marinescu 2017).
The effectiveness of e-commerce is an example of big data helping managers to provide services that suit clients’ needs (Addo-Tenkorang and Helo 2016; Yu et al. 2016). Traditional brick and mortar stores were impacted by the shift in online purchases because they did not have the same access to customer data (Stevens and Johnson 2016). However, the use of big data is not that limited, and some scholars found a way to expand its capabilities to the sphere of supply chain management.
In studies aiming to integrate big data into the supply chain research, the central term is big supply chain analytics. These analytics use data for calculations to determine the best activities and choices that the members of a supply chain need to make (Biswas and Sen 2016).
Although this form of predictive analysis is not new, the expansion of data sets beyond the traditional internal ERP and local supply chain management systems provides a more in-depth insight and more detailed information. Calculations used in big data are also advanced, employing statistical methods previously unavailable due to hardware and software restrictions (Giannakis and Louis 2016). As a result, the findings of such studies are beneficial to the decision-making process, making the operations in the supply chain reliable, effective and smooth.
To understand which elements of the supply chain can benefit from the application of big data, one has to present the standard design of a chain. First of all, the planning of sales, inventory and operations can be considered. This segment of the chain requires such analyses as supplier risk management, inventory projection and planning and forecasting optimisation (Gunasekaran et al. 2017).
All parts of the process rely on data, thus implying that the amount and quality of such information greatly determines the accuracy of the designed plans. In this case, the outcome of a successful estimation has to maintain the balance between the lack and the overabundance of goods – storing too much or not enough affects the ways in which a company builds relationships with its clients (Tan et al. 2015). By utilising big data, organisations can attempt to use real-time demand changes to shape their supply.
Moreover, in the process of planning, companies can influence prices by monitoring the mismatch between demand and supply numbers collected through big data. This can allow such activities as timing special offers, promotions, limited deals and new product campaigns (Giannakis and Louis 2016). Here, the information gathered from local sources would be less effective as it would represent only a small part of the client base.
The next process in supply chain management is sourcing, usually rooted in such calculations as supplier performance review and spend analysis. To source materials and services, an organisation has to model the costs to see what or who drives them, analyse the partner companies’ compliance with the contract and calculate the balance between demand and supply (Wang et al. 2016). Big data can improve all of these processes, acquiring information not only from internal operations but all available knowledge from the Internet. Delivery patterns of the partners, as well as their whole history of disruptions, strikes, bankruptcies and fires, reveal the stability and potential of sourcing agents (Witkowski 2017). For example, if a company cannot decide on the price quote for a product or service, it can source all available information about previous deals and model their price accordingly.
Production is another part of the supply chain – manufacturing needs to create timeframes, control the quality of goods, optimise procedures and account for the space that machinery can occupy. As can be seen, big data can offer many benefits to this element as well. For example, detailed analytics can reveal time frames when energy prices are at a low point, structuring the schedule to place the most energy-consuming processes into these periods (Addo-Tenkorang and Helo 2016).
This feature can be complemented by the use of artificial intelligence (AI) for processes’ automatization, leading to an increase in cost efficiency. By storing data such as parts’ dimensions and other information needed for manufacturing, computers can run root-cause analyses to identify ways to reduce defects (Singh et al. 2015). Overall, big data’s capability to collect and use large amounts of data is related to another cloud computing-based feature, the Internet of Things (IoT), which will be reviewed further.
The fourth element of supply chain management is warehousing. Here, one has to choose the best location for a warehouse, allocate spaces for products, pick the best positions for workers and choose routes to decrease the time of good’s movement from suppliers to their place of storage. Furthermore, the workload and costs need to be estimated and optimised. The use of logistics in this sphere of operations is traditional in the discipline, as some of the first studies were interested in improving storage approaches (Gunasekaran et al. 2017). Thus, warehousing-focused research has been using ERP data for years, with businesses investing in the latest equipment to optimise their facilities (Christopher 2016). The introduction of big data furthers the scholars’ efforts by expanding the amount of information that can be accessed for improvement.
In combination with other technologies, companies can now use data from cameras, forklift movement data and temperature and shelf weight sensors to create a full list of events that influence a warehouses’ results. By examining this information, one can see the route choices that people or machines make, estimate the number of errors in picking and shelving, and assess how driving behaviour can be changed to optimise all movements (Loy et al. 2016). Similar to the previous step in the supply chain, big data is essential in enhancing the root-cause analysis.
After being picked up, goods have to be moved to brick and mortar stores, retailer’s locations or consumers. Thus, transportation is another major element of a supply chain. The implementation of big data in this step is already in process – GPS navigation technologies are widely used by drivers to optimise the path from one location to another (Tian 2017). Fuel consumption statistics show which driving techniques, speeds, routes and other factors decrease costs and improve results (Mangan and Lalwani 2016). Real-time routing to determine the most efficient routes to destinations is based on traffic data, as well as trucks’ and clients’ locations (Witkowski 2017).
With the increase in data amounts, these calculations can become even more accurate, helping courier companies to save money on navigation and time on the road. Big data can also be used to support sustainable supply chain management. Such information as time periods when clients are most likely to be home or in the required location reduces the number of unsuccessful deliveries (Giannakis and Louis 2016). The choice of transport modes depending on the customer’s location and accessibility removes unnecessary movements, thus lowering carbon emissions and costs.
The next set of processes covers the chain’s point-of-sale operations. Here, data-driven decisions can be beneficial for both online and brick and mortar retailers. The configuration of stores (primarily offline) can determine consumer’s choices (Tan et al. 2015). Thus, information about clients’ patterns of picking goods from specific aisles and shelves is a category of big data analytics for this supply chain segment (Biswas and Sen 2016).
Many details of a purchasing instance can be considered using such technology, even if they do not connect with the quantity or quality of products directly. Some authors find that the examination of satellite images of store’s parking lots can predict businesses’ demand and help them stock inventory, anticipate sales, and determine the dates for special offers and sales (Wang et al. 2016). The monitoring of in-stock levels for goods can be a part of real-time restocking as well. All mentioned above data is further collected to anticipate demand fluctuations and determine the necessary amount of goods needed.
Artificial intelligence (AI)
The discussed above implementation of big data requires companies to invest in technologies that gather and examine a large amount of information. The decision-making step in these calculations is currently closely related to the integration of another cloud-based concept – artificial intelligence (AI). AI is a process in which machines perform processes similar to human intelligence, such as learning, analysis, reasoning and improvement, to reach decisions that are based on data and set standards (Pang et al. 2015). In business, the exploration of AI’s potential is closely tied with industries of healthcare, retail, and finance.
However, it also can be implemented in supply chain management as well. Scholars introduce three major directions in using AI in the supply chain, with the first one being a tool for sophisticated predictive analytics. As previously examined sources suggest, supply chain management relies substantially on forecasting – by estimating demand, managers determine further steps in procurement, transportation, restocking, and cost setting (Abdel-Basset et al. 2018). Therefore, the processes of examining previous data take up a large portion of the discipline’s necessary activities.
The amount of data available to businesses is vast, implying people’s inability to handle complex calculations by themselves. Even with the help of computer programmes, manual completion of predictions is challenging in large companies. Moreover, the results of such statistics are prone to human error (Zhou et al. 2015). As a result, the need for machine-operated support arises – platforms that are able to base their predictions with the help of big data and update the calculations automatically, adjusting for any existing influences are powered by AI (Ng et al. 2015). At the present time, some instances where AI is implemented for this purpose are considered in supply chain management scholarship.
One of the examples explored by researchers is software that uses big data to find underlying patterns in customer’s demand based on seasonal changes, location, preferences and other factors. Then, by analysing this information, the software can develop plans for a company, accounting for its goods’ specifics. For a furniture and home décor business, for instance, this platform can map out increases in demand during holiday seasons by noticing a pattern of increased décor-related purchases (Li and Li 2017).
Here, the importance of using AI is explained by the machine’s superior ability to record and use data from long-time periods automatically. Therefore, the concepts of big data and AI complement each other, and multiple steps in a supply chain become involved with new technology.
Sourcing, planning, manufacturing, and other stages are being equipped with AI software to map out the plan for future actions without the interference of people. Apart from the example of seasonal increases in demand, AI may also determine which of the products constitute the most popular (staple) items of a store’s assortment. This function is helpful to both offline and online stores, depending on their warehousing capabilities. The automation of these processes is explored by Kumar et al. (2017), who note that the ability of the machines to handle larger quantities of data impacts the outcomes of planning. Thus, the combination of AI and big data is integral to introducing cloud-based technologies to the supply chain.
A recent case of integrating AI into the supply chain in the pharmaceutical industry considers a German company, Merck. The company chose to use AI to automate its product distribution and materials’ allocation (Krittanawong et al. 2018). Merck installed a series of sensors as well as software for machine learning predictive analytics for that purpose. As a result, the business’ productivity increased considerably as the accuracy of predictions became higher than it was with human experts (Nash 2016). In this example, it is apparent that the introduction of AI and big data analytics is beneficial for the firm’s performance.
The next possible purpose of AI in the chain refers to the step of warehousing management. In the 1950s, storing facilities began using automated guided vehicles (AGVs), which transported goods with the help of physical assistance (unique mechanisms to navigate the machines) (Gnimpieba et al. 2015). The introduction of AI, however, eliminates the need for such assistance – new software has functions of mapping and localisation, determining where each AGV is situated and to what place it should go, also developing an efficient route (Abdel-Basset et al. 2018).
Here, another idea, the Internet of Things (IoT) can be linked to the increased interconnectedness of warehouse equipment and managing software (Abdel-Basset et al. 2018). Thus, there exist instances where AGVs handle goods on a fully-automated basis, and the potential of this application has to be considered. At the present time, the examples of AGV automation are limited, with some case studies offering a small glimpse into what can be done to automated transportation in the future.
Apart from using data for analysis and guiding vehicles, AI also has an opportunity to act as a communicator for businesses and customers. In particular, the segment of procurement requires many interactions between the managers and employees of the sales and marketing departments to determine which goods are needed (Abdel-Basset et al. 2018). The manual process of obtaining this information can be challenging in large organisations where the number of workers and the amount of inquiries is difficult for a person to document and fulfil. In this case, the AI’s function is to record data from people, retrieve information from databases, perform required operations and provide answers to any related questions.
For example, a chatbot may check the shipment status of a product, search for contract details and display prices without human assistance (Abdel-Basset et al. 2018). Thus, the goal of researchers and developers in using AI becomes apparent – learning software increases automation and allows large quantities of data to be processed quickly and efficiently.
The Internet of Things (IoT)
Similar to all other concepts that derived upon the creation of cloud computing, the Internet of Things (IoT) is a system that is closely connected to other elements of computerised operations – automated data collection, AI and online-distributed software. In fact, the IoT is a combination of various devices, machines, and software, as well as people and other objects, that contribute to information collection and transfer (Gunasekaran et al. 2016b).
The main idea behind this concept is that human-to-human and human-to-machine interactions no longer constitute the fundamental basis of all processes, meaning that other objects can participate and even determine the operability of whole systems (Gunasekaran et al. 2016a). To take an example from one’s everyday life, a person may use a device that is connected to a network of other applications which exchange information without the direct participation of the user. Smart homes are another idea based on the IoT – their concept implies that the ‘conversation’ happens between devices, while a person inhabiting the space puts limitations or regulates the preferences regarding these interactions.
The idea of the IoT, while introduced at the beginning of the twenty-first century, is still in the stage of development. There exist some facilities and personal homes that could be considered ‘smart’ – employing several devices that interact with each other without outside interference. Nevertheless, the level of automation in supply chain management is not sufficient for researchers to look at present examples (Kache and Seuring 2017). As a result, most scholars look at the perspectives that the IoT could offer by analysing the successes and failures of machines’ performance by themselves, including the effectiveness of AI, big data and other-cloud-based elements.
Major companies, however, are engaged in processes’ computerisation, thus making steps towards the introduction of the IoT into the supply chain. For example, Amazon fosters collaboration between robots and people in its warehouses – machines move through the shelves to find products for each order, reading QR codes and using Wi-Fi to connect to employees (Scheiber 2019). While it is unclear whether these machines demonstrate the implementation of AI or big data, their introduction to the company’s system is a move to a collaborative human-computer and computer-computer framework of the IoT.
Apart from finders, robots at Amazon also fulfil the roles of movers, transporting items from one area to another (Lee and Lee 2015). The automation of this process is an example of machines reducing the number of tasks previously performed by people.
It has to be noted that the potential of IoT is not limited to warehousing. Nonetheless, the majority of examples that are currently available to researchers for actual measurements in productivity are connected to this particular element of the supply chain. It is possible that works discussing real-life examples of the IoT being used in other areas exist, but it was challenging to find these sources. Thus, one has to conclude that these spheres of the IoT development are still on their first stages of theoretical research and predictions.
Cloud-Based Design and Manufacturing
The use of cloud-based technologies offers another strategy for designing facilities for businesses. 3D modelling that utilises big data analytics can create warehouses and manufacturing plants that are built with the purpose of maximising the efficiency of all employees and machines (Gunasekaran et al. 2016a). People designing facilities for such organisations use their expertise and previously collected data to determine which configurations will be the most beneficial for the performance. Machines can do the same with one difference – their ability to process large amounts of data increases their accuracy of prediction (Kache and Seuring 2017).
As an outcome, the cloud-based design offers tools for optimisation levels that are potentially unmatched to the skills of human beings (Kärcher et al. 2018). Logivations, a German company, offers such cloud-based software for layout planning, giving its clients a platform to design their warehouses using technology (Wu et al. 2016). Their argument for using machines is aligned with most scholars’ expectations of software’s efficiency and data use.
The access to data stored on the internet is not the only feature of cloud computing. Cloud-based software does not use up the space of local computers, and it does not have the same high demands as programs that have to be installed locally. Therefore, companies with limited hardware resources can use such software and not be constrained by their computers’ specifications (Shee et al. 2018). Here, another side of cloud computing is brought up – in contrast to applications and programmes that have to be installed manually, cloud-based services do not require the same level of support and memory storage.
In comparison to other elements of cloud computing services, design programmes are not as researched or discussed in contemporary supply chain management scholarship. However, the potential of these applications has to be acknowledged as they integrate AI and big data analytics into several significant steps of the supply chain and produce results that can be further used to analyse efficiency and optimise processes. Cloud-based design software is another direction in which companies are currently moving.
Predictions for the Future of Cloud Computing in Business
A major part of the existing scholarship is devoted to investigating how cloud-based computing and the services that it can provide can affect the sphere of supply chain management. The details on each of the elements are described above; however, authors often consider them in a unified framework. According to Tjahjono et al. (2017), the innovation of the IoT and its incorporation into the supply chain will lead to the fourth industrial revolution which will significantly change the ways in which businesses operate. This process’ result is also called Industry 4.0, implying that the use of cloud-based technologies is a natural progression of evolution in manufacturing (Hashem et al. 2015). It is clear that the scholars expect the revolution to make the computerisation of supply chain processes a new standard of practice for companies.
The primary expectation for Industry 4.0 is a substantial shift in operations on all levels of the supply chain. The prediction of complete supply chain automation is made by many authors who expect manufacturing plants and warehouses to undergo radical changes in the next decades (Zhong et al. 2016). Researchers highlight the need to implement all services offered in the cloud simultaneously, using big data, AI, design and the IoT as parts of the whole.
As a result, the appearance and growth of smart factories are expected to start soon. In these facilities, the majority of all operations will be performed by machines with minimal human interaction. Therefore, apart from completing simple tasks such as pick-up and transportation, stationery computers and robots will use data to make decisions related to production and distribution and solve problems that arise in the factory.
The basic principles of the fourth industrial revolution are based on production systems networking, horizontal structures, engineering support and continuous acceleration. The first point implies that people and machines will interact in Cyber-Physical Systems (CPSs) to optimise processes (Ahmed et al. 2019). The vertical design of smart production systems implies that manufacturing facilities will be developed to be flexible and prepared for change (Gunasekaran et al. 2016a).
Thus, factories will be ready to respond to clients’ demands and even allow for advanced customisation. All data that is in any way connected to the processes in the supply chain will be recorded in the system. This will allow one to detect any problems and discrepancies and ensure full transparency of each action. The second principle is horizontal integration – new chain networks that include supply chain members, business partners and clients (Chang et al. 2016a). This type of system requires transparency, which can be achieved through the IoT and increased traceability of equipment present in the cloud.
The third principle is through-life support that is executed across the entire chain. Since innovation is a constant process in the cloud-based services and related hardware, engineering assistance for all members of the supply chain is key to keeping all elements up to date with technical improvements (Wenzel and Van Quaquebeke 2018). The engineering support is also vital to keeping big data available for the supply chain management. Finally, the last point in Industry 4.0 is the continuous change that happens due to exponential technologies. Self-learning AI constantly improves its operations and conclusions based on the acquired data (Mahdavinejad et al. 2018). Therefore, new processes appear and replace older strategies, increasing supply chains’ flexibility and customisation options for customers.
The combination of these principles demonstrates which particular concerns and hopes are brought up in contemporary supply chain research. It is clear that optimisation remains the central focus of all studies, meaning that the introduction of machines is explained by their ability to improve processes through automation. However, a large portion of the research aims to create a system that will integrate all solutions, while current cases of small changes are not considered as a vital part of the industrial revolution.
Research Methodology
The present work conducts exploratory research and employs data synthesis as the primary process for further analysis. The study aims to use the existing scholarship in the field of cloud computing and supply chain management. The purpose of data gathering is to present a summative view of how cloud computing is and can be used in supply chains. The scope of knowledge available for consideration demonstrates to what extent scholars and managers understand cloud computing and its potential for business. The synthesis of qualitative and quantitative studies presented in this work was completed using a thematic analysis.
The approach of thematic analysis is appropriate for the study because its central goal is to identify themes of meaning drawn from the existing scholarship (Bell et al. 2018). The search criteria included academic articles in English that contained such terms as “cloud computing”, “Internet of Things”, “big data”, “cloud-based design” and “supply chain”. During the data collection process of the study, 70 academic works were examined to gather information about the different spheres of cloud computing and their implementation in the supply chain. The employed methodology allowed the author to draw information from research articles and case studies that considered the topics of cloud computing, IoT, big data, AI and cloud-based design.
The selection of works was based on the aim of locating several patterns that would explain which themes are prevalent in current research of supply chain management and Industry 4.0. Furthermore, the contents of such discussions were investigated to summarise the notions that authors propose regarding this topic. The direction in information gathering and analysis is presented below (Figure 1).
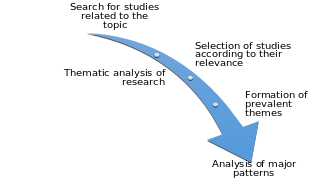
As a result of examining the existing scholarship and completing the thematic analysis, major topics were identified in research regarding the implementation of cloud computing in the supply chain. First of all, the implications of current use are presented in a number of studies that focus on current examples and their effectiveness in improving the performance of supply chains. Next, a number of studies choose to offer some perspectives and scenarios on how cloud computing and its elements will improve the supply chain in the future. Here, the attention is shifted from real-life examples toward the theoretic models for integration as well as technology that is on its first stages of development. Third, a large portion of the scholarship raises concerns that may plague the supply chain under the influence of the industry 4.0. In this case, most studies either highlight the potential benefits of this industrial revolution or discuss the risks that this change entails.
The investigation of the articles showed that authors often considered similar issues and posed the same questions as the result of their research. For instance, such works as the article by Saarikko et al. (2017) and the study by Thames and Schaefer (2016) discuss the same inevitable process of cloud computing significantly changing the supply chain. Thus, the thematic analysis shows the prevalence of such patterns as “current use”, “perspectives”, “potential benefits” and “potential risks”.
While many of the researched sources included several of these themes, their distinction allows one to see which major topics were the most investigated by scholars and which particular predictions and analyses are similar across the studies. The following analysis presents the synthesis of information collected from the chosen articles and case studies, discussing both the real-life examples provided by the researchers and their implications for academic research.
Analysis
Current Use
The research concerned with current examples of cloud computing being used in the supply chain is widely discussed in the literature review. The cases that discuss the companies making steps in the implementation of cloud computing share a particular pattern – they concentrate on particular services noting that the existing efforts cannot be viewed as a full installation of Industry 4.0. For example, the case of Merck, a German company that introduced AI into its product distribution facilities, states that this feature does not eliminate the need of people to complete some operations, thus showing the limited integration of the IoT (Nash 2016). Here, the use of big data is mentioned briefly, and the focus is kept on small actions and procedures that were changed with the help of machines.
Similarly, the case of Logivations, a German firm that offers cloud-based design software for warehousing and manufacturing facilities, concentrates on the use of programmes by organisations as a singular scenario that views this opportunity as a small step that does not assuredly lead to major innovation. The discrepancy between theoretical and practical models presented in the literature demonstrate the lack of fully developed solutions to offer an entire system for a supply chain where each element would be connected to the IoT and cloud-based technologies (Tiwari et al. 2018; Syntetos et al. 2016). Nonetheless, a significant part of these articles offers some perspectives, which expand on the small steps, expecting major changes to come in some ways.
Perspectives
The primary expectation of the scholarship in regards to implementing cloud computing into the supply chain is the start of the fourth industrial revolution and the subsequent emergence of Industry 4.0. First of all, full interoperability of all elements in the supply chain implies that machines and sensors installed by the company work in a single network, sharing data with each other and the people who enter the system through CPSs (Ahmed et al. 2019). This can be achieved if all vehicles, robots, computers and other devices become a part of the IoT structure.
The examples of companies taking steps towards Industry 4.0 demonstrate that such interoperability has not been achieved. In the case of Amazon, many robots are used to transport or find goods, but since only a small number of facilities have this system, the inconsistency in the contribution and documentation between different locations lowers the interoperability of the organisation. Amazon’s complex structure incorporates multiple steps of a supply chain. Thus, it is possible to expect further automation, and researchers note that the use of robots in a number of facilities does not create a system of full synergy between people and computers expected from Industry 4.0-level organisations.
In Merck’s example, the potential for a fully automated supply chain is discussed. The managers of the firm note that this idea is not a part of the immediate action plan, but a concept for the future of the organisation (Nash 2016). Nonetheless, it can be implied from the research that the scholars see this principle of industrial innovation as the most achievable. The majority of studies views interoperability as possible to reach in the next decades since some steps are being taken to create the IoT structure.
Furthermore, since large corporations and small businesses are increasing their rates of automation, the idea that an interconnected system will be the standard appears to be realistic (Attaran and Woods 2018). It is unclear how soon will all members of the supply chain reach this level of innovation or whether they will be able to implement the new structures at the same time.
The second principle is information transparency – sensors installed by the company to collect data and upload it to a database for future use by other members of the network. The data collected by devices is then turned into a fully digitalised copy of all systems and facilities of the business, making analyses and predictions accurate and based on information that updates in real-time (Ali et al. 2017). Apart from that, the access to this information makes it transparent – all data is stored in one cloud, and different professionals, facilities, and stakeholders can use it to improve the processes (Zhou et al. 2018).
Furthermore, the concept of transparency is analysed from the perspective of customers and partner companies. In transportation, for instance, the location of goods and the speed and route of vehicles carrying them are pieces of information that buyers and sellers want to know (Thakur and Gunnlaugsson 2018). Thus, firms can install sensors that record this information and upload it to the cloud to create a map or a log of all movements. They may also use AI to estimate the date of delivery or predict any problems with the transportation process.
The issue of information transparency is also brought up due to increasing environmental concerns. The concept of sustainability is now a part of all responsibilities that businesses have to acknowledge. Clients want to know how their goods are made and delivered to them – cloud computing provides them with an opportunity to look into the operations of the automated companies (Chang et al. 2016b). By using AI and transporting the data to the cloud, businesses also lower the consumption of physical goods – hardware and materials that would be engaged in the supply chain (Sarkis 2019).
Green manufacturing and Industry 4.0 are connected in their aim to lower energy consumption and make products using less tangible and intangible resources (Castillo et al. 2018). Information transparency is expected to increase clients’ awareness of this change and make companies more stable on the market.
Technical assistance is the third principle in establishing Industry 4.0. Here, the relationship between humans and machines is investigated, establishing that technology in newly improved facilities will perform two main functions. The first one is that of a data-provider in which machines use predictive analytics to supply human workers with visualised information for making decisions about complex or urgent problems (Verdouw et al. 2016).
Computers have the ability to present data in a comprehensible way, reducing large amounts of numbers and calculations to graphs and critical points (Chen and Zhao 2018). The use of big data is such analyses requires the adaptation of the results due to people’s limited capabilities of understanding information. Thus, technical assistance is a way to keep people in the network while reducing the pressure on them participating in analytic processes.
Another role that machines have is more physical – devices are able to perform actions that are dangerous, difficult, exhausting or simply unpleasant for people. Robots that move through facilities, as in Amazon’s example, lower workers’ need to walk considerable distances from one product to another (Scheiber 2019). As such warehouses can take up vast spaces, the use of technology can be beneficial not only to the company but to people’s health as well (Lindqvist and Neumann 2017). In transportation, robots can decrease the hazards of accidents or eliminate the problem of fatigue for drivers and machinery operators (Saarikko et al. 2017).
This principle, apart from offering many benefits for organisations, also may lead to some drawbacks for workers and clients, which will be discussed later. Machines already work with hazardous materials and perform routine operations in manufacturing. Thus, this part of the principle is easy to achieve for companies on the basis of existing examples. Nonetheless, if one accounts for the need of these devices to be assistants in intellectual labour as well, the completion of the instructional revolution seems distant.
The final principle that demonstrates scholars’ expectations for the supply chain is decentralisation. The introduction of AI and big data into the supply chain logistics is expected to increase the autonomy of machines in making decisions on all stages of the chain (Li et al. 2017). The potential of this change, however, is also supplemented by the fact that AI can be self-learning and self-improving. Thus, it is assumed that machines will eventually be able to solve more complex problems without human assistance. The independence of the devices from human interaction increases their control over the manufacturing and other operations (Ben-Daya et al. 2017).
As a result, the idea of decentralised decisions is brought up. Machines that are fully capable of completing the cycle of the supply chain do not have a singular agent responsible for decision making but a series of software programmes, the capabilities of which correspond to the appropriate step. Here, the authors’ vision is guided by the expectations of full automation.
At the current stage of development, warehouse operating software has the potential of introducing fully self-learning facilities that use predictive analytics for managing inventory storage. The example of Amazon, where people currently restock shelves and monitor the picking robots, and their interaction with transporting robots can be reviewed. In a fully automated facility, the use of sensors and cameras directed at shelves with the goods will allow the software to determine whether restocking is necessary (Lee et al. 2018; Reaidy et al. 2015).
Furthermore, this decision will depend on the machine’s use of big data analytics to predict whether a particular product will be in high demand in the season (Mahdavinejad et al. 2018; Mostafa et al. 2019). In this case, the interference of a human worker is not needed, and delegation to people is performed as an exception when computers are malfunctioning or facing an unsolvable dilemma.
As one can see, the principles of Industry 4.0 serve as the main directions in which scholars are expecting the supply chain innovation to proceed. Thus, such perspectives as full automation, robotisation of processes, and the decreasing role of human workers are among the central ideas that researchers discuss in their paper, often using resent cases of large companies as a foundation for future improvements.
Potential Benefits
From the research of the current and future applications of cloud computing and cloud-based technology, it becomes apparent that these concepts can provide many advantages to the sphere of supply chain management. The thematic analysis demonstrates that studies most often consider the benefits of particular programmes and methods that become available in the cloud, including the IoT, big data and AI. Thus, the following analysis will also consider these systems separately.
The benefits of big data lie both in the amount of information and the ways to analyse and use it. Big data can be characterised by three significant descriptors – volume, variety and velocity (Figure 2). The volume describes the ability of computers to store, transfer and examine large amounts of data – an action that is too challenging for people to perform without assistance. The digitisation of sources previously available only in print form creates a data log for past information, while the currently virtual recording of information on the Internet adds to the continuously growing pool.
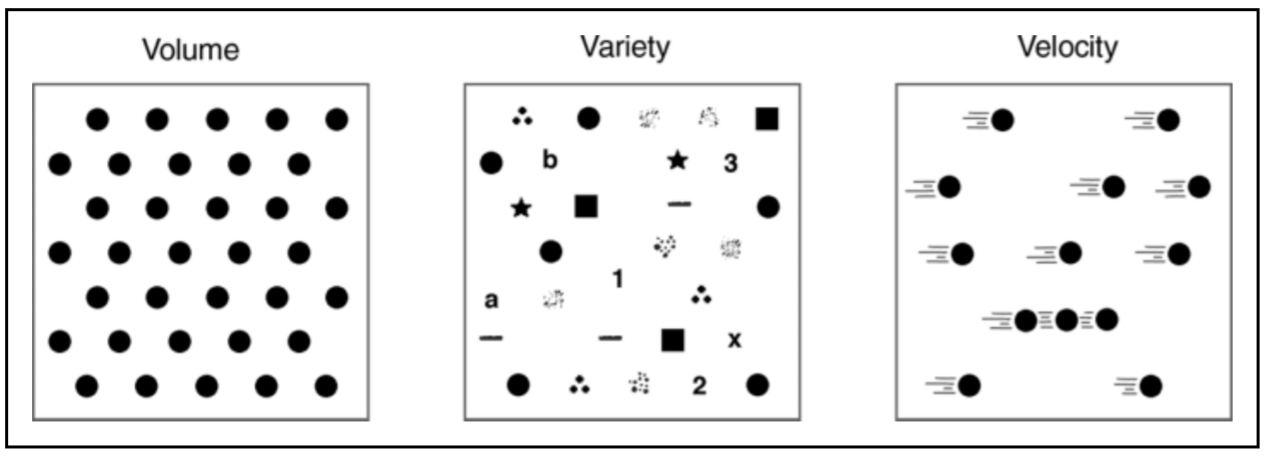
Big data is a method of harnessing the knowledge from these recordings. Variety is a concept that describes the heterogeneity of data in content and form – videos, pictures and other types of media, search queries, posts, clickstreams and user interaction with an application are all a part of big data (Wenzel and Van Quaquebeke 2018). Thus, algorithms analysing this information have a variety of sources from which to draw knowledge, thus making the search for patterns possible in virtually any topic. Finally, velocity describes the speed of data accumulation on the Internet. With millions of people using various online and offline services every day, the amount of information available for analysis in enormous.
Each of these characteristics provides its own opportunities to supply chain management. The volume of data increases the accuracy of population descriptions for analytical tools (Raguseo 2018). This feature factors in the company’s understanding of target audiences, including people’s preferences, demands, and interests (Chen et al. 2015). As a result, big data allows one to build representative samples that maximises the efficiency of predictions.
The volume also helps one to discover patterns in a massive cesspool of data (Huong Tran et al. 2016). A small number of data points does not guarantee the detection of systematic influences or fluctuations, while large amounts of information increase models’ granularity (Wenzel and Van Quaquebeke 2018). This factor is beneficial for observing behavioural patterns and enhancing their investigations for further improvement. Finally, volume leads to more discoveries previously unavailable to researchers. Big data’s outreach is substantial, recording every bit of information and tying it to the rest of the available knowledge. Thus, such interconnectedness of data grants new opportunities to planners and managers in many areas of the supply chain.
Variety decreases the influence of bias in analytics, allowing to arrive at a concrete and evidence-based conclusion. Singular experiments, questionnaires, conversations, and records can provide the company with different results which may be influenced by both researchers and responders (Wenzel and Van Quaquebeke 2018). In contrast, the variety of data analysed by computers at the same time can lower the impact of such factors, leading to results that represent people’s choices and other information in a less biased way.
Moreover, as people do not always answer truthfully or behave how they want to behave in social situations, data inferred from one source cannot create the full picture of an event. Big data overcomes this problem by harnessing information from several channels, locating discrepancies and affording a more faithful measure (Wenzel and Van Quaquebeke 2018). As a result, big data’s variety offers one a perspective that would be impossible to view without the help of machines.
The use of AI in works is frequently combined with the discussion of the IoT, thus implying that the benefits of machine learning are often included with the overall advantages of the IoT in the supply chain. Furthermore, as the IoT is a system that encompasses many programmes and analytical methods, its studies also discuss the outcomes of implementing cloud-based design and big data. The introduction of the IoT to different elements of the chain leads to a variety of changes.
Mostafa et al. (2019) propose a number of enhancements that the IoT could bring to the industry (Figure 3). On the first stage, demand planning, IoT devices are engaged in data collection and analysis (big data), which results in the development of accurate and efferent demand forecasts. The collection of information without big data happens manually, providing a small and unreliable sample size for further analysis.
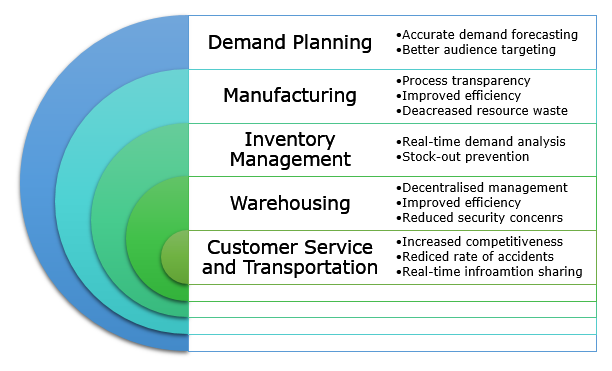
In manufacturing, the IoT is implemented to increase the transparency of each step, thus providing more data to the stakeholders and increasing the potential for sustainability and social responsibility. Furthermore, the scalability of processes is improved due to machine-performed calculations. Big data analytics help preserve resources by calculating the most efficient way to produce goods.
For instance, the IoT-operated factory may conserve energy by working without lights and using electricity at night when the stations have a lower workload (Haddud et al. 2017). The inventory management and warehousing benefits are substantial – the combination of predictions and real-time analysis lowers the chance of stock out (Juntao and Yinbo 2016). Robots locate products, share their location thought the cloud, pick them up and choose the best route for delivery enhance the effectiveness of the facility. Moreover, the need for hiring staff also diminishes, which results in financial savings (Jayaraman et al. 2018). Security problems can also be eliminated by smart sensors and real-time information-sharing.
The use of the IoT in transportation can benefit the communication between customers, shippers and carriers by including them and computerised support into one system for interaction. Chatbots described as an opportunity for replacing customer support in solving simple problems are believed to reduce waiting times for answers and improve the accuracy of answers, as bots will be able to access and analyse the data quickly (Kache and Seuring 2017).
The flexibility and transparency of the services are also expected to improve due to the amount of real-time information being shared with all parties. In this case, the main strength of the IoT is its contribution to sustainability (Wu et al. 2017). Clients interested in green manufacturing will view be interested in having access to the company’s operations and movements.
The financial benefit of reducing the staff and conserving resources can further help businesses grow and expand their reach or focus on niches that are the most profitable (according to AI-managed analytics). As a result, the combined benefits of spending less and being sustainable will further distinguish smart factories and business in the market (Haddud et al. 2017). Overall, the scalability and interconnectivity of such facilities will result in a supply chain with high synergy and effectiveness. As a result, these features increase the competitiveness of a business designed according to the principles of Industry 4.0.
Potential Risks
Every innovation poses risks to the industry’s efficiency and safety, and Industry 4.0 is not an exception. In the case of automation, the first potential threat is related to the application of big data analytics without considering which information should be prioritised for demand predictions (Ivanov et al. 2019). The amount of available data in the global network is both an advantage, and a drawback for calculations – the lack of quality filters can lead one to false conclusions and predictions that will not align with actual demand (Clarke 2016).
The outcome of such incorrect calculations can be substantial – a business can experience stockouts, increased waste of resources, customer dissatisfaction and loss of the main client base (Clarke 2016). Thus, it is vital to pay attention to the implementation of big data analytics on its first stages and create a system of standards for data selection. Self-learning machines are likely to adjust their settings according to the discrepancies in predicted and real demand (Clarke 2016). Nonetheless, these changes will require time, possibly impacting the business’ performance for some time.
The lack of trust in the IoT technologies is another problem that some companies may experience. If an organisation’s top management or its leading professionals do not accept the new structure, their resilience to its adoption will lead to conflicts, limited access of computers to information, and lost potential of using the IoT (Zsidisin and Henke 2019). The confidence in the new system has to be established in order to increase employees’ participation and stakeholders’ preparedness to work in the framework.
One more risk arises if people unwilling to work with the IoT lower the productivity of the facility. It is clear that the increased automation will affect the job market and lower the number of engaged workers on every step of the supply chain (Saarikko et al. 2017). Therefore, companies with a large number of employees that can make substantial cuts may be met with a negative response from both workers and customers. Here, one may suggest redistributing staff, offering training where possible and working to reduce the resistance to change in order to improve the performance.
The costs of implementation can become an issue if the organisation incorrectly estimates the budget for new devices and systems. If a firm chooses to make significant changes to its structure, especially if it is not automated, it may risk losing productivity or creating a system that is unreliable and exposed to cyber threats (Brous et al. 2019). Moreover, the installation of new technology requires time for adjustment, and one’s lack of preparation to face possible consequences of systems’ ineffectiveness can lead to further financial losses.
The overreliance on technology is not a problem on its own, but other issues can render highly-automated businesses completely inactive. Unstable internet connection, power outage, natural disasters, viruses and malware and other outside interventions are a risk that is out of one’s control (Chasaki and Mansour 2015). These dangers can affect any plant or firm, regardless of their size or level of innovation, but their impact on fully autonomous facilities can be devastating to resources, productivity and business’ financial stability.
The lack of standard architectures on the current stage of the IoT development poses a risk to companies that operate in industries with conflicting market forces. As devices in the IoT structure often require some set of rules before they start to perform calculations or make decisions, the inexistence of reliable guidelines can result in such network being incapable of making correct choices (Brous et al. 2019). Companies often need to design their own technologies to align with their objectives and markets. Thus, their professionals must have sufficient knowledge in the field of the IoT and cloud computing, or the development of the new system will be impossible.
Legal frameworks for managing information in public and private spaces are also underdeveloped. This means that companies introducing advanced technologies to their system can encounter security concerns (Brous et al. 2019). International and local laws do not offer a robust framework for using big data or protecting businesses from an information breach. While some laws are in place, the inability to address all arising concerns in this field poses significant risks to companies. Data privacy conflicts initiated by both corporate entities and individuals may result in one experiencing reputational damage (Brous et al. 2019). Legal action against one’s use of data is possible as well, thus urging companies to establish systems which will prevent unnecessary disclosure of private data.
For instance, purchasing data obtained by firms that use them for demand prediction is a valuable addition to big data analytics, but it is also a piece of information that exposes one’s private information (Boyes 2015). On the one hand, businesses need this knowledge to improve their services. On the other hand, people may object to providing this data due to their personal beliefs or anxieties. Thus, the legality of obtaining and using private information remains a grey area that is not defined by string standards for all cases.
Overall, the problem of data privacy is discussed as one of the most hazardous and challenging in the field of cloud computing. The benefits of interconnectivity and transparency make information more accessible, but also imply that customers and business’ private information can be misused and manipulated by outside actors. Companies that increase their participation in the IoT network also expose themselves to security threats. Currently developed IoT devices contain serious vulnerabilities which cybercriminals can use to their advantage (Boyes 2015). The risks of inadequate (or non-existent) encryption, software protection and lacking authorisation security make the whole structures open to attacks.
This risk is exceptionally high for services that are responsible for the security of information, facilities and other software. If a hacker gains access to these programmes, the whole organisation can be at risk. One of the possible solutions to lower the danger of cyberattacks is developer training to teach them how to implement encryption and protection into the software (Davis 2015). Furthermore, knowledge about IoT security features has to be distributed to companies choosing to undergo industrial change (Davis 2015).
Special programmes such as firewalls, intrusion prevention, and backup channels have to be utilised to limit the vulnerability of data. The model for cybersecurity of supply chains has been developed by Boyes (2015), who suggests that such elements as trustworthiness and continuity are vital to preserving the safety of data (Figure 4).
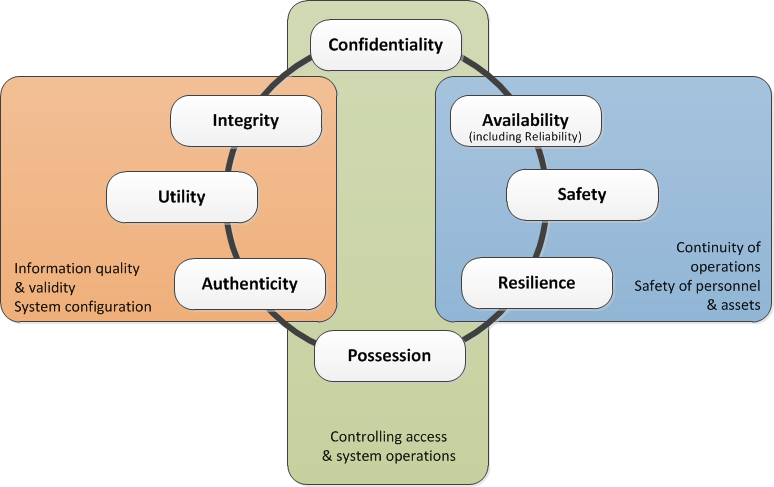
The perspective of continuity increases the availability of assets and safety of operations – disruptions in the continuity increase the risk of private data escaping the closed system. The aspects of possession and confidentiality are derived from establishing who or what can have access to the system (Boyes 2015). Finally, the quality of information that is used by the chain’s IoT is ensured by its authenticity, utility and integrity (Boyes 2015). Together, these characteristics create a framework that is supported by protective systems and high-quality data as well as clear distinctions between persons and machined with or without control.
Conclusions
Supply chain management is a discipline that heavily relies on the latest innovations and people’s demands. Through its history, one can see that all modifications to the supply chain have been made to increase the productivity and efficiency of each step. The computerisation of the chain was a significant step that changed the way scholars and managers view supply chain elements and their interactions. Another move that is currently considered in related scholarship is the fourth industrial revolution that will result in the establishment of Industry 4.0. This era of supply chain management is discussed with cloud computing at the foundation, arguing that automation of processes is the next logical step necessary to meet the demands of the growing population.
The aim of this research was to examine the current and potential impact of cloud computing and its elements on the supply chain. Such solutions as big data analytics, AI, IoT, and cloud-based design were identified during the data collection process, and their united and separate contribution to the industry was considered. Thematic analysis utilised as the method for interpreting selected sources revealed a number of patterns in the existing scholarship. Major themes included the current use of cloud computing and its services, perspectives for future use as well as benefits and risks related to the introduction of cloud computing to the chain.
Big data is a method of collecting and analysing the amounts of information that cannot be handled by humans without the help of machines. Previously, the volume of data available to companies was limited to their own networks’ databases and written or locally recorded customer information. Analytical tools using data from the cloud gain access to a diverse set of media and client’s history of interaction with various businesses. As a result, the calculations using this data can be more accurate and insightful.
Artificial intelligence (AI) further enhances the effectiveness of these analytics, equipping devices with machine learning and increasing their ability to manage processes without the supervision of humans. AI’s implementation in the supply chain furthers the development of Industry 4.0 guided by the principles of technological assistance and decentralised decision-making. With the use of AI, human-to-human and computer-to-human systems are complemented a computer-to-computer structure that allows factories and other facilities to operate with full automation.
Finally, the system of devices that collect and transfer data and use it in different parts of the supply chain creates the IoT. The combination of cloud-powered services leads to the development of a network in which machines and people share and use information in real time, increasing the control of the business over each object and process of the organisation. IoT is a driver of the Industry 4.0 principle of interconnection.
According to the research, the integration of cloud computing and its services offers many benefits to the supply chain. Automated manufacturing, transportation, demand planning and other elements of the chain are expected to be accurate, flexible, customisable, sustainable, and resilient. The competitiveness of companies that develop and maintain these qualities is high, and the continuous improvement of machines can further the growth of profit, while also increasing the efficiency and reducing resources use.
However, it is vital to realise the risks of automation, such as overreliance on technology, outside threats, resistance to change, job loss, sustainability concerns, and legal problems. Cybersecurity is among the primary dilemmas in the field since cloud computing remains largely vulnerable to cybercrimes. More research is necessary to design systems for Industry 4.0 that will be safe and effective for customers, governments and businesses. However, cloud computing has the potential to change the supply chain substantially, and its integration has already started to impact the world.
This research has demonstrated the current state of the integration of cloud computing in the supply chain and shown that, while the ideas of Industry 4.0 are expanding their influence on small and large businesses, only a small number of prominent corporations were able to move towards the goals of the fourth industrial revolution. Moreover, although scholars have broadly researched the risks that cloud computing poses to the security of information, the lack of a legal system controlling these processes reveals the unpreparedness of the world to fully embrace cloud computing as a part of the supply chain.
Bibliography
Abdel-Basset, M. et al., 2018. Internet of Things (IoT) and its impact on supply chain: a framework for building smart, secure and efficient systems. Future Generation Computer Systems, 86, pp. 614-628.
Addo-Tenkorang, R. and Helo, P.T., 2016. Big data applications in operations/supply-chain management: a literature review. Computers & Industrial Engineering, 101, pp. 528-543.
Ahmed, M.U. et al., 2019. Micro-foundations of supply chain integration: an activity-based analysis. Logistics, 3(2), p. 12.
Ali, M.M. et al., 2017. Supply chain forecasting when information is not shared. European Journal of Operational Research, 260(3), pp. 984-994.
Almarabeh, T. and Majdalawi, Y.K., 2019. Cloud computing of e-commerce. Modern Applied Science, 13(1), pp. 27-35.
Attaran, M. and Woods, J., 2018. Cloud computing technology: a viable option for small and medium-sized businesses. Journal of Strategic Innovation and Sustainability, 13(2), pp. 94-106.
Bell, E. et al., 2018. Business research methods. 5th ed. Oxford: Oxford University Press.
Ben-Daya, M. et al., 2017. Internet of things and supply chain management: a literature review. International Journal of Production Research, pp. 1-24.
Biswal, A.K. et al., 2018. Warehouse efficiency improvement using RFID in a humanitarian supply chain: implications for Indian food security system. Transportation Research Part E: Logistics and Transportation Review, 109, pp. 205-224.
Biswas, S. and Sen, J., 2016. A proposed architecture for big data driven supply chain analytics. ICFAI University Press (IUP) Journal of Supply Chain Management, 13(3), pp. 7-34.
Boyes, H., 2015. Cybersecurity and cyber-resilient supply chains. Technology Innovation Management Review, 5(4), pp. 28-34.
Brous, P. et al., 2019. The dual effects of the Internet of Things (IoT): a systematic review of the benefits and risks of IoT adoption by organizations. International Journal of Information Management, pp. 1-17.
Castillo, V.E. et al., 2018. Supply chain integrity: a key to sustainable supply chain management. Journal of Business Logistics, 39(1), pp. 38-56.
Chang, V. et al., 2016a. Cloud computing adoption framework: a security framework for business clouds. Future Generation Computer Systems, 57, pp. 24-41.
Chang, V. et al., 2016b. Organisational sustainability modelling — an emerging service and analytics model for evaluating cloud computing adoption with two case studies. International Journal of Information Management, 36(1), pp. 167-179.
Chasaki, D. and Mansour, C., 2015. Security challenges in the internet of things. International Journal of Space-Based and Situated Computing, 5(3), pp. 141-149.
Chen, F. et al., 2015. Data mining for the internet of things: literature review and challenges. International Journal of Distributed Sensor Networks, 11(8), p. 431047.
Chen, J. and Zhao, W., 2018. Logistics automation management based on the Internet of things. Cluster Computing, pp.1-8.
Christopher, M., 2016. Logistics & supply chain management. 5th ed. London, UK: FT Publishing.
Clarke, R., 2016. Big data, big risks. Information Systems Journal, 26(1), pp. 77-90.
Davis, A. 2015. Building cyber-resilience into supply chains. Technology Innovation Management Review, 5(4), pp. 19-27.
Diaby, T. and Rad, B.B., 2017. Cloud computing: a review of the concepts and deployment models. International Journal of Information Technology and Computer Science, 9(6), pp. 50-58.
Giannakis, M. and Louis, M., 2016. A multi-agent based system with big data processing for enhanced supply chain agility. Journal of Enterprise Information Management, 29(5), pp. 706-727.
Gnimpieba, Z.D.R. et al., 2015. Using Internet of Things technologies for a collaborative supply chain: application to tracking of pallets and containers. Procedia Computer Science, 56, pp. 550-557.
Gunasekaran, A. et al., 2017. Big data and predictive analytics for supply chain and organizational performance. Journal of Business Research, 70, pp. 308-317.
Gunasekaran, A. et al., 2016a. Flexible sustainable supply chain network design: current trends, opportunities and future. Global Journal of Flexible Systems Management, 17(2), pp. 109-112.
Gunasekaran, A. et al., 2016b. Information technology governance in Internet of Things supply chain networks. Industrial Management & Data Systems, 116(7), pp. 1-31.
Haddud, A. et al., 2017. Examining potential benefits and challenges associated with the Internet of Things integration in supply chains. Journal of Manufacturing Technology Management, 28(8), pp. 1055-1085.
Hashem, I.A.T. et al., 2015. The rise of “big data” on cloud computing: review and open research issues. Information Systems, 47, pp. 98-115.
Hong, P. et al., 2017. Global markets, innovation eco-system and technology infrastructure development: global supply chain perspective. Perspectives on Global Development and Technology, 16(5), pp. 483-486.
Huong Tran, T.T. et al., 2016. Supply chain information sharing: challenges and risk mitigation strategies. Journal of Manufacturing Technology Management, 27(8), pp. 1102-1126.
Ivanov, D. et al., 2019. The impact of digital technology and Industry 4.0 on the ripple effect and supply chain risk analytics. International Journal of Production Research, 57(3), pp. 829-846.
Jayaraman, R. et al., 2018. Internet of Things and the future of manufacturing industry. Indian Journal of Computer Science, 3(3), pp. 7-25.
Jede, A. and Teuteberg, F., 2015. Integrating cloud computing in supply chain processes: a comprehensive literature review. Journal of Enterprise Information Management, 28(6), pp. 872-904.
Juntao, L. and Yinbo, M., 2016. Research on internet of things technology application status in the warehouse operation. International Journal of Science, Technology and Society, 4(4), pp. 63-66.
Kache, F. and Seuring, S., 2017. Challenges and opportunities of digital information at the intersection of Big Data Analytics and supply chain management. International Journal of Operations & Production Management, 37(1), pp. 10-36.
Kang, Y.S. et al., 2015. MongoDB-based repository design for IoT-generated RFID/sensor big data. IEEE Sensors Journal, 16(2), pp. 485-497.
Kärcher, S. et al., 2018. Sensor-driven analysis of manual assembly systems. Procedia CIRP, 72, pp. 1142-1147.
Krittanawong, C. et al., 2018. Big data, artificial intelligence, and cardiovascular precision medicine. Expert Review of Precision Medicine and Drug Development, 3(5), pp. 305-317.
Kumar, V. et al., 2017. Developing a framework for traceability implementation in the textile supply chain. Systems, 5(2), p. 33.
Kushida, K.E. et al., 2015. Cloud computing: from scarcity to abundance. Journal of Industry, Competition and Trade, 15(1), pp. 5-19.
Lamba, K. and Singh, S.P., 2017. Big data in operations and supply chain management: current trends and future perspectives. Production Planning & Control, 28(11-12), pp. 877-890.
Lee, C.K.M. et al., 2018. Design and application of Internet of things-based warehouse management system for smart logistics. International Journal of Production Research, 56(8), pp. 2753-2768.
Lee, I. and Lee, K., 2015. The Internet of Things (IoT): applications, investments, and challenges for enterprises. Business Horizons, 58(4), pp. 431-440.
Li, B. and Li, Y., 2017. Internet of things drives supply chain innovation: a research framework. International Journal of Organizational Innovation, 9(3), pp. 71-92.
Li, B.H. et al., 2017. Applications of artificial intelligence in intelligent manufacturing: a review. Frontiers of Information Technology & Electronic Engineering, 18(1), pp. 86-96.
Liao, Y. et al., 2017. Past, present and future of Industry 4.0 – a systematic literature review and research agenda proposal. International Journal of Production Research, 55(12), pp. 3609-3629.
Lindqvist, U. and Neumann, P.G., 2017. The future of the Internet of Things. Communications of the ACM, 60(2), pp. 26-30.
Loy, M.L. et al., 2016. Beyond the use of robotics: operations and supply chain control for effective inventory management in a health system pharmacy. In A. GUPTA, et al., eds., Advances in healthcare informatics and analytics (pp. 145-155). Cham, Switzerland: Springer.
Maccarthy, B.L. et al., 2016. Supply chain evolution – theory, concepts and science. International Journal of Operations & Production Management, 36(12), pp. 1696-1718.
Mahdavinejad, M.S. et al., 2018. Machine learning for Internet of Things data analysis: a survey. Digital Communications and Networks, 4(3), pp. 161-175.
Mangan, J. and Lalwani, C.L., 2016. Global logistics and supply chain management. 3rd ed. Chichester, UK: John Wiley & Sons.
Marinescu, D.C., 2017. Cloud computing: theory and practice. 2nd ed. Cambridge, MA: Morgan Kaufmann.
Monczka, R.M. et al., 2015. Purchasing and supply chain management. 6th ed. Boston, MA: Cengage Learning.
Mostafa, N. et al., 2019. Impacts of Internet of Things on supply chains: a framework for warehousing. Social Sciences, 8(3), p. 84.
Nash, K.S., 2016. Merck deploys AI for ‘self-driving’ supply chain. New York, NY: The Wall Street Journal. Web.
NG, I. et al., 2015. Contextual variety, Internet-of-Things and the choice of tailoring over platform: mass customisation strategy in supply chain management. International Journal of Production Economics, 159, pp. 76-87.
Pang, Z. et al., 2015. Value-centric design of the internet-of-things solution for food supply chain: value creation, sensor portfolio and information fusion. Information Systems Frontiers, 17(2), pp. 289-319.
Qin, J. et al., 2016. A categorical framework of manufacturing for industry 4.0 and beyond. Procedia CIRP, 52, pp. 173-178.
Raguseo, E., 2018. Big data technologies: an empirical investigation on their adoption, benefits and risks for companies. International Journal of Information Management, 38(1), pp. 187-195.
Reaidy, P.J. et al., 2015. Bottom-up approach based on Internet of Things for order fulfillment in a collaborative warehousing environment. International Journal of Production Economics, 159, pp. 29-40.
Roblek, V. et al., 2016. A complex view of industry 4.0. Sage Open, 6(2), pp. 1-11.
Saarikko, T. et al., 2017. The Internet of Things: are you ready for what’s coming? Business Horizons, 60(5), pp. 667-676.
Sarkis, J., ed., 2019. Handbook on the sustainable supply chain. Cheltenham, UK: Edward Elgar Publishing.
Scheiber, N., 2019. Inside an Amazon warehouse, robots’ ways rub off on humans. New York, NY: The New York Times. Web.
Shee, H. et al., 2018. The impact of cloud-enabled process integration on supply chain performance and firm sustainability: the moderating role of top management. Supply Chain Management: An International Journal, 23(6), pp. 500-517.
Singh, A. et al., 2015. Cloud computing technology: reducing carbon footprint in beef supply chain. International Journal of Production Economics, 164, pp. 462-471.
Stevens, G.C. and Johnson, M., 2016. Integrating the supply chain… 25 years on. International Journal of Physical Distribution & Logistics Management, 46(1), pp. 19-42.
Syntetos, A.A. et al., 2016. Supply chain forecasting: theory, practice, their gap and the future. European Journal of Operational Research, 252(1), pp. 1-26.
Tan, K.H. et al., 2015. Harvesting big data to enhance supply chain innovation capabilities: an analytic infrastructure based on deduction graph. International Journal of Production Economics, 165, pp. 223-233.
Tao F. et al., 2018. Data-driven smart manufacturing. Journal of Manufacturing Systems, 48, pp. 157-169.
Thakur, M. and Gunnlaugsson, V.N., 2018. Information sharing strategies in whitefish supply chains in Norway vs. Iceland: impact on supply chain decision making. International Journal on Food System Dynamics, 9(3), pp. 240-252.
Thames, L. and Schaefer, D., 2016. Software-defined cloud manufacturing for industry 4.0. Procedia CIRP, 52, pp. 12-17.
Tian, F., 2017. A supply chain traceability system for food safety based on HACCP, blockchain & Internet of things. IEEE 2017 14th International Conference on Service Systems and Service Management, pp. 1-6.
Tiwari, S. et al., 2018. Big data analytics in supply chain management between 2010 and 2016: insights to industries. Computers & Industrial Engineering, 115, pp. 319-330.
Tjahjono, B. et al., 2017. What does industry 4.0 mean to supply chain? Procedia Manufacturing, 13, pp. 1175-1182.
Verdouw, C.N. et al., 2016. Virtualization of food supply chains with the internet of things. Journal of Food Engineering, 176, pp. 128-136.
Waluyo, W., 2019. Does computerized accounting system increase the supply chain accuracy? An empirical evidence from Indonesian supply chain companies. Uncertain Supply Chain Management, 7(3), pp. 541-552.
Wang, G. et al., 2016. Big data analytics in logistics and supply chain management: certain investigations for research and applications. International Journal of Production Economics, 176, pp. 98-110.
Wenzel, R. and Van Quaquebeke, N., 2018. The double-edged sword of big data in organizational and management research: a review of opportunities and risks. Organizational Research Methods, 21(3), pp. 548-591.
Witkowski, K., 2017. Internet of things, big data, industry 4.0 – Innovative solutions in logistics and supply chains management. Procedia Engineering, 182, pp. 763-769.
Wu, K.J. et al., 2017. Toward sustainability: using big data to explore the decisive attributes of supply chain risks and uncertainties. Journal of Cleaner Production, 142, pp. 663-676.
Wu, L. et al., 2016. Smart supply chain management: a review and implications for future research. The International Journal of Logistics Management, 27(2), pp. 395-417.
Yu, Y. et al., 2016. E-commerce logistics in supply chain management: practice perspective. Procedia CIRP, 52, pp. 179-185.
Zhong, R.Y. et al., 2015. A big data approach for logistics trajectory discovery from RFID-enabled production data. International Journal of Production Economics, 165, pp. 260-272.
Zhong, R.Y. et al., 2016. Big Data for supply chain management in the service and manufacturing sectors: challenges, opportunities, and future perspectives. Computers & Industrial Engineering, 101, pp. 572-591.
Zhou, L. et al., 2015. Supply chain management in the era of the internet of things. International Journal of Production Economics, 159, pp. 1-3.
Zhou, Z. et al., 2018. The persuasive and informative effects of information disclosure: evidence from an online supply chain finance market. New York: SSRN. Web.
Zsidisin, G.A. and Henke, M., eds., 2019. Revisiting supply chain risk. Cham, Switzerland: Springer.